PharmacoEEG research trend in mental health care
- Dae Keun Kim
- 2024년 11월 18일
- 4분 분량
EEG Study Predicts Medication Response in ADHD, The frontal alpha excess group responded 87% to the antidepressant, and the frontal theta excess group responded 100% to the stimulant. (Suffin & Emory, 1995)
Pharmaco EEG study in MDD, 1 in 7 people with EEG-based medication selection showed improvement, but only 1 in 6 people without EEG-based medication selection showed clinical improvement.(Stephen C Suffin et al., 2007)
In a study looking at responsiveness to SSRIs used in MDD, those with a higher alpha theta ratio (ATR) in the prefrontal lobe responded better to SSRI, while those with a lower ATR responded better to dopamine+norepinephrine. (Leuchter et al., 2009)
Serotonin may affect alpha sources in the thalamo-cortico-thalamic loop to adjust anterior-posterior alpha gradient and dopamine may stimulate mesocortical neural circuits to decrease theta and increase beta. (Fingelkurts & Fingelkurts, 2022; Steriade, Gloor, Llinás, Lopes da Silva, & Mesulam, 1990)
In Clinical EEG and Neuroscience, 11 EEG phenotypes were presented that can guide clinical intervention. No specific phenotype was matched to a specific psychiatric problem, but rather the phenotypes were found to be common to a variety of psychiatric problems, and appropriate interventions were suggested for each phenotype.. (Gunkelman, 2014; Johnstone, Gunkelman, & Lunt, 2005)
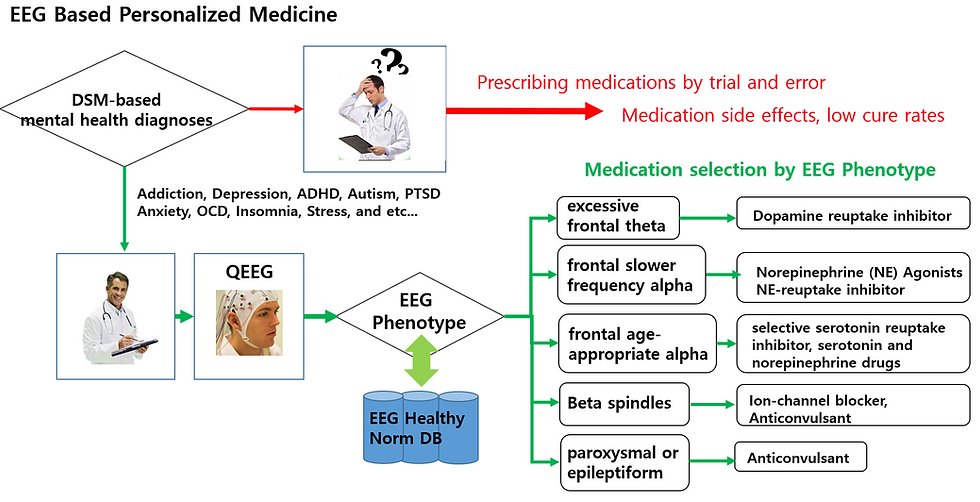
Fig. 1 EEG-based Medication Prediction Concepts(Gunkelman, 2014)
Examining data from 386 refractory psychiatric patients with schizophrenia, anxiety, autism, and attention deficit disorder, four phenotypes were observed: focal slowing, beta spindle, transient discharge, and encephalopathy. They found that prescribing a drug that did not match the phenotype led to the development of refractory cases, with strong implications for the use of phenotype-matched drugs in the future.(R. J. Swatzyna, Kozlowski, & Tarnow, 2015; Ronald J. Swatzyna et al., 2024)
The excessive beta/beta spindles subtype, which accounts for 13-20% of ADHD, did not respond to the stimulant, while the excessive theta subtype did. Those with a paroxysmal pattern responded to anticonvulsants, while those with ACC-origin theta activity did not respond to antidepressants. (Olbrich, van Dinteren, & Arns, 2015)
In the STAR-D study, which compared best-advised prescribing with prescribing based on rEEG (quantitative electroencephalography based on a database of healthy people), patients who received rEEG prescribing reported statistically significantly greater improvement in symptoms from week 2 to week 12 (DeBattista et al., 2011), and the need to utilize computational psychiatry was suggested in Nature neuroscience.(Huys & Maia, 2016)
The most readily available and undervalued biomarker in psychiatry is EEG/QEEG, which is also highly reliable given its relatively short measurement time. The strongest and most repeated predictors of treatment response in depression come from EEG compared to sMRI or fMRI. EEG signals such as individual alpha peak frequencies, arousal metrics, and machine-learning derived multivariate EEG patterns have been repeatedly shown to predict treatment outcomes in depression, PTSD, ADHD, addiction, and more. EEG is already approved for clinical use by the FDA in areas such as epilepsy diagnosis, polysomnography, depth of anesthesia testing, and neurofeedback. Given the current urgency for biomarkers in psychiatry, EEG is cost-effective compared to fMRI and the technology is innovative enough to be collected at home, but the overwhelming bias of most studies toward fMRI needs to be reconsidered.(Etkin & Mathalon, 2024)
References
DeBattista, C., Kinrys, G., Hoffman, D., Goldstein, C., Zajecka, J., Kocsis, J., . . . Fava, M. (2011). The use of referenced-EEG (rEEG) in assisting medication selection for the treatment of depression. J Psychiatr Res, 45(1), 64-75. doi:10.1016/j.jpsychires.2010.05.009
Etkin, A., & Mathalon, D. H. (2024). Bringing Imaging Biomarkers Into Clinical Reality in Psychiatry. JAMA Psychiatry, 81(11), 1142-1147. doi:10.1001/jamapsychiatry.2024.2553
Fingelkurts, A. A., & Fingelkurts, A. A. (2022). Quantitative Electroencephalogram (qEEG) as a Natural and Non-Invasive Window into Living Brain and Mind in the Functional Continuum of Healthy and Pathological Conditions. Applied Sciences, 12(19), 9560. Retrieved from https://www.mdpi.com/2076-3417/12/19/9560
Gunkelman, J. (2014). Medication Prediction with Electroencephalography Phenotypes and Biomarkers. Biofeedback (Online), 42(2), 68-73. doi:https://doi.org/10.5298/1081-5937-42.2.03
Huys, Q. J., & Maia, T. V. (2016). Computational psychiatry as a bridge from neuroscience to clinical applications. 19(3), 404-413. doi:10.1038/nn.4238
Johnstone, J., Gunkelman, J., & Lunt, J. (2005). Clinical database development: characterization of EEG phenotypes. Clin EEG Neurosci, 36(2), 99-107. doi:10.1177/155005940503600209
Leuchter, A. F., Cook, I. A., Gilmer, W. S., Marangell, L. B., Burgoyne, K. S., Howland, R. H., . . . Greenwald, S. (2009). Effectiveness of a quantitative electroencephalographic biomarker for predicting differential response or remission with escitalopram and bupropion in major depressive disorder. Psychiatry Res, 169(2), 132-138. doi:10.1016/j.psychres.2009.04.004
Olbrich, S., van Dinteren, R., & Arns, M. (2015). Personalized Medicine: Review and Perspectives of Promising Baseline EEG Biomarkers in Major Depressive Disorder and Attention Deficit Hyperactivity Disorder. Neuropsychobiology, 72(3-4), 229-240. doi:10.1159/000437435
Steriade, M., Gloor, P., Llinás, R. R., Lopes da Silva, F. H., & Mesulam, M. M. (1990). Basic mechanisms of cerebral rhythmic activities. Electroencephalography and Clinical Neurophysiology, 76(6), 481-508. doi:https://doi.org/10.1016/0013-4694(90)90001-Z
Suffin, S. C., & Emory, W. H. (1995). Neurometric subgroups in attentional and affective disorders and their association with pharmacotherapeutic outcome. Clin Electroencephalogr, 26(2), 76-83. doi:10.1177/155005949502600204
Swatzyna, R. J., Kozlowski, G. P., & Tarnow, J. D. (2015). Pharmaco-EEG: A Study of Individualized Medicine in Clinical Practice. Clin EEG Neurosci, 46(3), 192-196. doi:10.1177/1550059414556120
Swatzyna, R. J., Morrow, L. M., Collins, D. M., Barr, E. A., Roark, A. J., & Turner, R. P. (2024). Evidentiary Significance of Routine EEG in Refractory Cases: A Paradigm Shift in Psychiatry. Clinical EEG and Neuroscience, 15500594231221313. doi:10.1177/15500594231221313
[1] Sequenced Treatment Alternatives to Relieve Depression (STAR*D) was a collaborative study on the treatment of depression, funded by the National Institute of Mental Health.
Comments